Rapid mapping of tephra fallout building damage: A machine learning approach
Presentation
Authors: Tennant, E., Jenkins, S., Miller, V., Wen, B., Robertson, R.
Event: Cities on Volcanoes
Summary: This conference presentation proposes an automated approach leveraging unmanned aerial vehicle acquired optical imagery to expedite the assessment of post-eruption building damage.
Explosive volcanic eruptions generate tephra fallout that poses a significant threat to buildings in the surrounding area. Following an eruption, the rapid identification of affected structures and the quantification of damage severity are paramount for effective recovery planning. Traditional building damage surveys, typically conducted on the ground by trained personnel, can be labor-intensive and time-consuming.
To expedite the assessment of post-eruption building damage, we propose an automated approach leveraging unmanned aerial vehicle (UAV) acquired optical imagery collected after the 2021 eruption of La Soufrière volcano (St Vincent nd the Grenadines) and state-of-the-art convolutional neural networks (CNNs). Our comprehensive machine learning framework encompasses all necessary models and pre-processing steps for:
1) Building Extraction: our framework accurately extracts buildings from background imagery;
2) Damage Classification: It efficiently distinguishes between undamaged and damaged buildings, achieving a robust F1 score of 95% on the St Vincent test dataset;
3) Severity Assessment: Our framework further categorises damaged buildings into moderate damage and major damage categories, with an F1 score of 74% on the test dataset.
The spatial data generated by our framework enables the rapid generation of precise building damage maps which may serve as valuable resources for guiding decision-makers in post-eruption recovery management.
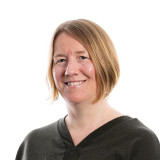
Victoria Miller
Project Co-Leader: Mitigate